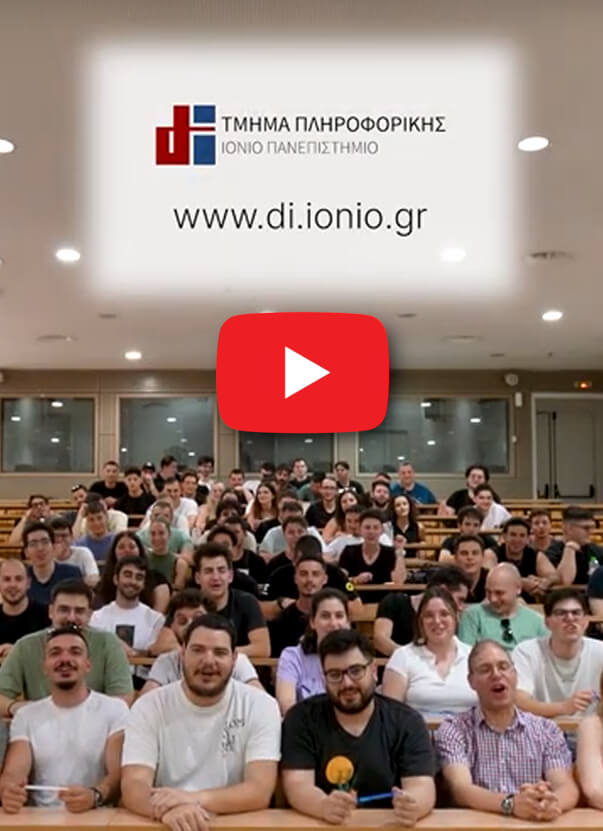
A message from the Head of Department of Informatics
The Department of Informatics of the Ionian University is the only department in Greece adopting an interdisciplinary association of ICT in the humanities. The Department offers a modern curriculum that is constantly updated on the basis of developments in computer science and research achievements of faculty members.
Students can practice their theoretical knowledge in thematic workshops related to important computer science subjects such as databases and information systems, bioinformatics and neuroinformatics, security and computational modeling. It is worth mentioning that many graduates of the Department continue their studies at postgraduate and doctoral level in academic institutions in Greece and abroad.
Studies
01 |
Undergraduate StudiesΤο Τμήμα Πληροφορικής του Ιονίου Πανεπιστημίου έχει ως αντικείμενο τόσο τη θεωρητική, όσο και την εφηρμοσμένη Πληροφορική και προσφέρει συστηματική και υπεύθυνη πανεπιστημιακή διδασκαλία | |
02 |
Postgraduate StudiesΤο Τμήμα Πληροφορικής του Ιονίου Πανεπιστημίου έχει ως αντικείμενο τόσο τη θεωρητική, όσο και την εφηρμοσμένη Πληροφορική και προσφέρει συστηματική και υπεύθυνη πανεπιστημιακή διδασκαλία | |
03 |
Doctroal StudiesΤο Τμήμα Πληροφορικής του Ιονίου Πανεπιστημίου έχει ως αντικείμενο τόσο τη θεωρητική, όσο και την εφηρμοσμένη Πληροφορική και προσφέρει συστηματική και υπεύθυνη πανεπιστημιακή διδασκαλία |